- Caveminds: 7-9 Figure Founder AI Community
- Posts
- Founder Insights from Million-Dollar LLM Projects to the Rise of the RAG AI Strategy [Part 1]
Founder Insights from Million-Dollar LLM Projects to the Rise of the RAG AI Strategy [Part 1]
From the Limitations of LLMs to the Promise of RAG – Your Comprehensive Guide to Navigating the AI Landscape. Let's explore The Future of AI: Boundless Possibilities with LLMs.

In today’s Deep Dive…
The Studio that Startups and Enterprises use to integrate RAG into their businesses
Maximize Your AI ROI with Retrieval Augmentation Generation
Golden Nuggets of Wisdom: Unlocking the Full Potential of LLMs
The Future of AI: Exploring the Boundless Possibilities of LLMs
Strategies to Boost LLM Efficiency and Accuracy
Making Your AI Transparent and Trustworthy
Seize the Moment or Fade: Andrew & Ben's Urgent Call to Action on RAG AI — Caveminds Podcast
Hey there, this is Andrew from NineTwoThree Studio.
Today’s Deep Dive will highlight part 1 of 2 of our learnings from building million-dollar budget AI projects featuring an LLM for Fortune 500 and startup companies.
In this special edition, you’ll want to watch this special Caveminds Podcast episode and read this deep dive to 10X the impact.
Let’s dive in…
Read Online and listen to the audio version.
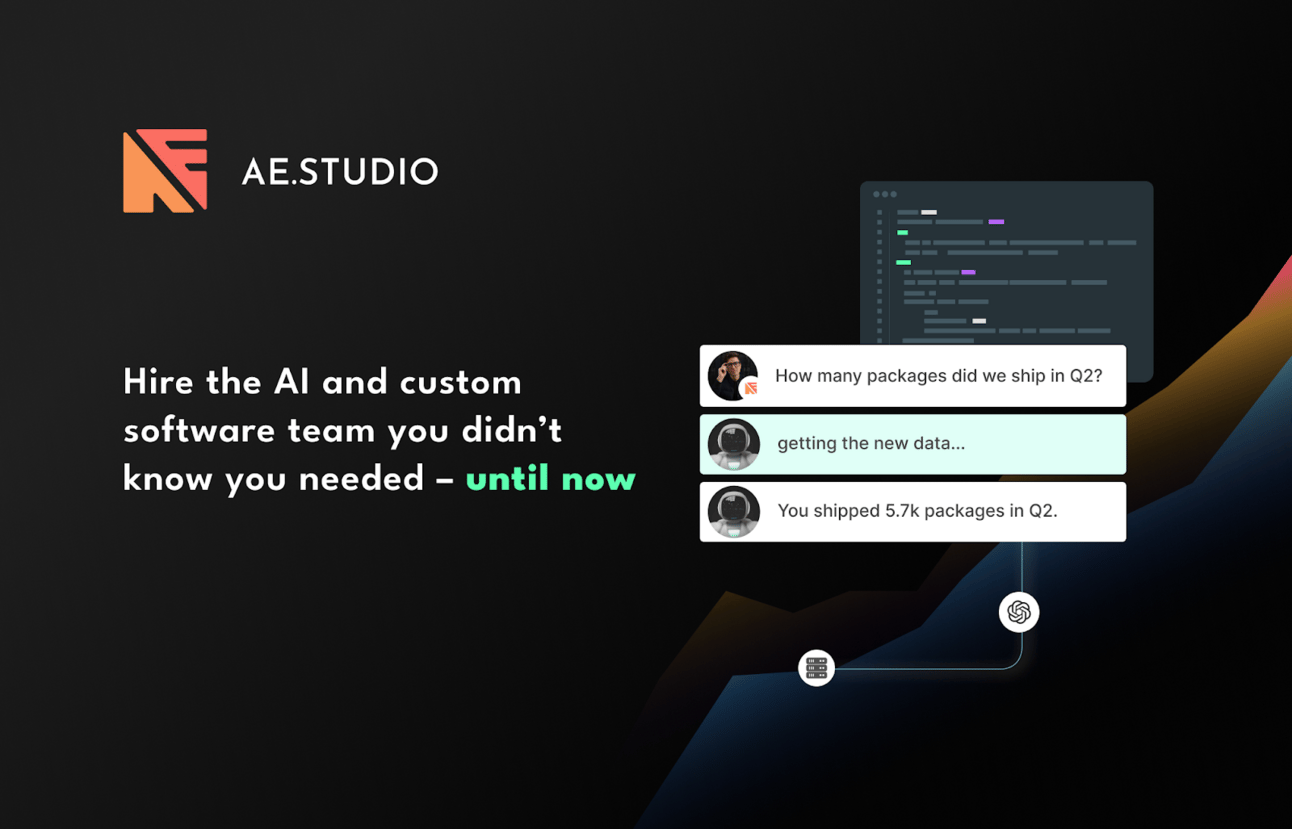
How do you implement the right AI strategy for your product?
Hire AE Studio's world-class team of software builders to craft and implement the optimal AI solution for your business. — Yes, even RAG systems.
Our development, design, and data science product studio works closely with founders and executives to create custom software, machine learning, and BCI solutions.
From custom-built MVPs to bespoke AI/ML solutions, see how you can leverage AI to achieve your business objectives.
Join 9,000+ founders getting actionable golden nuggets that are tailored to make your business more profitable.

DEEP DIVE OF THE WEEK

How Retrieval Augmented Generation Powers Enterprise Generative AI Projects
Large Language Models (LLMs) have taken the spotlight since the release of ChatGPT.
LLM’s can perform a wide range of contextual tasks at almost the same level of competence as a human.
From Google Translate using LLMs to improve the fluency of its translations to Grammarly providing more comprehensive and informative feedback on users’ writings, we have seen almost every tool we use daily come out with its own LLM implementation.
To the untrained AI practitioner, it could seem that launching an LLM is an easy task.
As with any groundbreaking technology, Generative AI has imperfections.
One of the most prominent of these limitations is the challenge faced by LLMs in delivering accurate, sourced, and contextually appropriate content.
This main limitation makes it almost impossible for a company to stake their reputation based on an LLM’s unknown output.
How would you feel if a brand you trusted lied to you while you asked them questions about your account?
This fear has led to the inception and growing relevance of retrieval-augmented generation (RAG). With RAG, companies have more control over the materials an LLM uses for its information and the answers it gives users.
So first, we will dive into the challenges with LLMs.
Challenges with Large Language Models (LLMs):
Why companies cannot trust using LLMs:
LLMs do not know relevant information
LLMs do not state facts & often hallucinate.
LLMs do not provide the source link.
One of the most significant associated with LLMs is the inability to control what information it uses to generate responses.
Oftentimes the LLM will even hallucinate by generating factually incorrect, nonsensical text.
Hallucination generally happens for the following reasons:
Incomplete or contradictory training data,
Lack of common sense,
Lack of context.
(We highly encourage you to check our full article on hallucinations and what steps you can take to detect and overcome it!)
But to understand why hallucinations exist, we need to take a step further into the foundational model by first clarifying Generative AI - then using these building blocks to introduce RAG as the framework for enterprise-grade applications.
What is a Foundational Model ?
Foundational models are a form of generative artificial intelligence that generates output from one or more inputs, which we call prompts, in the form of human language instructions.
These AI models are trained on large datasets of existing data, and they learn to identify the patterns and structures within that data.
Once trained, the model can generate new data that is similar to the data it was trained on, but not identical.
But it's just a story, a series of random words generated from a request. We can see how bias inserts itself into a foundational model with the example below.
Explaining Bias in the Foundational Model

A simple explanation of why models hallucinate is to understand how they are trained.
Let’s start with a piece of knowledge that was accepted by all humans before but was later disproved.
How many planets are in our solar system?
The foundational model was trained on thousands of textbooks, of all reputable sources that explained that Pluto is in fact a planet.
Since 2006, however, there are a plethora of blogs, articles and books that explain why Pluto is no longer a planet.
Asking ChatGPT this question yields the following answer:

The response is accurate and factual. However, let’s ask a second question:

What is happening here?
How can the foundational model understand Pluto is not a planet, but still mixing up facts from when we believed it was a planet?
All foundational models have human bias. That is, the employees at OpenAI have trained the results to be presented in a certain way.
In this example, humans trained the model during its training stage to state that Pluto is not a planet.
But there was no supervision to explain if Pluto is gaseous or rock.
Over the years, astronomers have determined that Pluto is more gaseous than originally expected and therefore, much lighter.
The LLM is left to rationalize the information it has both before and after 2005 and proudly stated false information.
Until enough people ask the question and thumb down the response - or OpenAI employees adjust the tuning - the model will always confuse this “fact.”
It is important to provide the facts from a trusted source. But each company's trusted source is different and we cannot rely on the “facts” from foundational models because foundational models are inherently biased.
Continue reading this Deep Dive and get full access to our AI Intelligence platform, getting access to:
✔️ The full AI Deep Dives library
✔️ Monthly AI Webinar Workshops
✔️ Premium Podcast Exclusive Guides
✔️ Private Slack Channel for Entrepreneurs
✔️ Monthly AI Strategy Playbooks With Video Walkthroughs
✔️ Monthly Live AI Events & Meetups
✔️ Quarterly AMA’s With Caveminds Founding Team
✔️ And much more…
Subscribe to Premium to read the rest.
Become a paying subscriber of Premium to get access to this post and other subscriber-only content.
Already a paying subscriber? Sign In.
A subscription gets you:
- • Premium Weekly AI Strategy Deep Dives
- • AI Deep Dive Library
- • Monthly AI Webinar Workshops
- • Premium Podcast Exclusive Guides
Reply